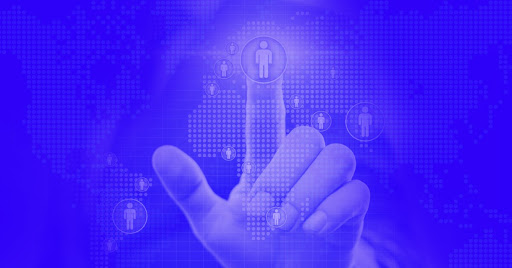
According to Accenture statistics, AI will boost profitability by 38% and generate $14 trillion of additional revenue by 2035. Using product recommendations gives approx. 500% higher conversions compared to the generic offer. There are not many credible sources describing how the various recommendation engines work and which algorithms are more effective, hence we chose to compare them, including non-AI and AI-based offers to find out which one is the most efficient.
Types and examples of product recommendations
Product recommendation engines are systems that enable displaying personalized, compliant with each client’s product information to increase conversion rate and sales. Recommendations can be displayed in a variety of ways such as landing pages, e-mail notifications, or web push. We can distinguish 3 types of product recommendations:
- Product-based – Recommendations depend on the product attributes
- 1-to-1 – Recommendations depend on the individual user actions.
- AI – Recommendations depend on the similarity between the user and other users.
Our goal was to find out how each type of AI and non-AI recommendations work in generating revenue and how their efficiency is compared with the one of mass communication. The conducted research has given us an updated picture of the AI and non-AI recommendations, allowing us to show the most effective recommendation types.
We took into account the whole spectrum of data of several dozens of e-commerce vendors utilizing dynamic and AI recommendations in their strategy and conducted a set of statistical analyzes aimed at determining the effectiveness of each of the tested types of recommendations.
Product recommendations delivery channels analysis
One of the areas which we wanted to recognize after the research, was the impact of the recommendation delivery method on the results. To make the comparison accurate, we concentrated on the communication channels that allow the determination of both, display and click in the recommended products.
We included:
- mass email campaigns
- mass web push campaigns
- automated email campaigns
- automated web push campaigns
Recommendations in automated web pushes generate the highest revenue
We start our study with the analysis of click rate in recommendations from different sources – automated and mass emails and web pushes. According to our research:
- Automatic messages generate more revenue than mass messages
- Bulk web pushes generate more revenue per click than mass mailing and workflow
- Automatic web pushes generate more revenue than automatic emails
Web push notifications with product recommendations surpass the emails in effectiveness and generated revenue.
The results can be seen on the graph below:
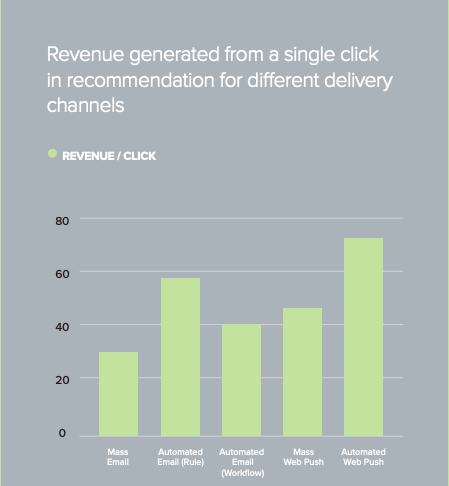
Effectiveness of different recommendation types
After conducting the above research, we wanted to check if there is a difference in the effectiveness of different recommendation types. Is AI guaranteeing better conversion compared to other recommendation types?
We checked how the efficiency varies for the most popular recommendation algorithms both AI and non-AI-based capabilities to deliver personalized offers and found out that AI-based algorithms, in general, prove to accomplish higher conversion and precision than non-AI recommendation types. However, the highest precision can be reached by using a mix of the two mentioned above.
What is the best recommendation engine?
As AI recommendations are becoming a standard in the modern eCommerce world, many companies offer their solutions leaving marketers with a troublesome task to choose the most effective one.
According to research (Comparative Evaluation of Top-N Recommenders in e-commerce: an Industrial Perspective) that evaluates well-known recommendation algorithms, the effectiveness of SALESmanago’s recommendations is positioned on the top.
Comparison of precision for different recommendations engines:


Precision is a metric used in the evaluation of prediction models. It responds to the question: What proportion of positive identifications was actually correct. In the case of recommendation algorithms, it shows how many customers’ purchases, we are able to find at least one of the recommended products.
SALESmanago’s AI recommendations engine outperforms other solutions available on the market in the precision of the product recommendations by reaching a 5.2 times higher average.
If you want to know more about product recommendation benchmarks take a look at the full research.